Machine learning (ML) continues to revolutionize industries and redefine how we interact with technology. As we move further into 2025, the rapid evolution of machine learning trends offers exciting opportunities for businesses, developers, and researchers alike. From ethical learning models to real-time AI, these trends are not just buzzwords—they are shaping the future of automation, decision-making, and human-computer interaction.
In this article, we’ll explore the most impactful machine learning trends of 2025, with insights into how they are being applied across various industries.
1. Real-Time Machine Learning Takes the Lead
One of the most significant machine learning trends in 2025 is the rise of real-time processing. Unlike traditional batch-based models, real-time ML systems adapt and respond instantly to new data. This capability is crucial for applications in finance, cybersecurity, and e-commerce, where decisions must be made in milliseconds.
Additionally, the integration of edge computing allows ML models to function closer to the source of data, reducing latency and improving efficiency. As a result, companies are increasingly investing in real-time machine learning for fraud detection, predictive maintenance, and customer personalization.
2. Ethical and Explainable AI Becomes a Priority
Transparency in machine learning has become a global concern. In response, machine learning trends are shifting toward models that are both ethical and explainable. Stakeholders now demand to understand how decisions are made, especially in sectors like healthcare and law enforcement.
To address this, developers are incorporating explainable AI (XAI) techniques that break down complex model decisions into understandable elements. Moreover, regulatory bodies are pushing for AI ethics frameworks, encouraging organizations to build ML systems that avoid bias and ensure fairness.
3. Industry-Specific Machine Learning Applications
Another prominent development is the emergence of specialized machine learning models tailored to specific industries. These custom-built solutions outperform general-purpose models because they understand domain-specific language, patterns, and behaviors.
For instance, in the healthcare sector, ML algorithms are being used to predict patient readmission rates and personalize treatment plans. Meanwhile, in agriculture, machine learning models analyze satellite imagery to optimize crop yields. Clearly, machine learning trends now point toward deeper integration within individual verticals, enhancing both accuracy and performance.
4. Generative Models Gain Mainstream Adoption
Generative models such as GANs (Generative Adversarial Networks) and transformers have taken center stage in the ML world. In 2025, these tools are more powerful and accessible than ever. They can generate high-quality text, images, audio, and even video content, making them invaluable in entertainment, education, and design.
Not only do generative models fuel creativity, but they also automate repetitive content production tasks, saving time and money. Importantly, organizations are now using machine learning to create synthetic data for training models—an approach that significantly enhances privacy and data diversity.
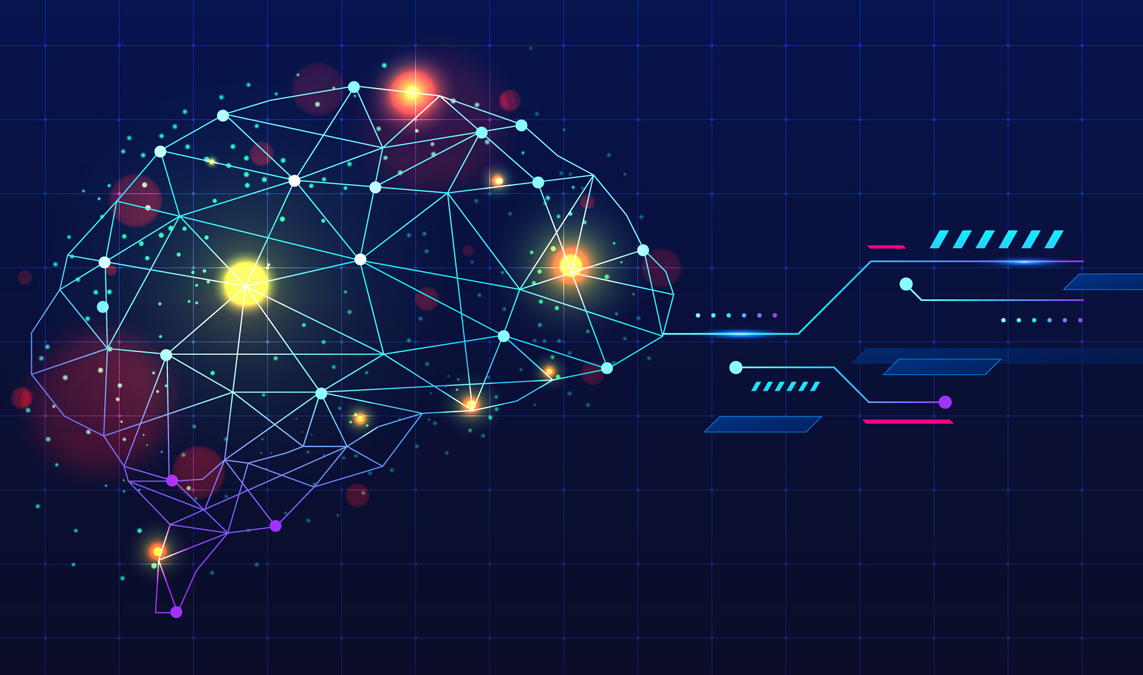
5. Automated Machine Learning (AutoML) Expands Accessibility
Previously, building an effective ML model required deep expertise. However, AutoML platforms are now one of the fastest-growing machine learning trends in 2025. These tools automate tasks such as feature selection, model selection, and hyperparameter tuning.
As a result, more businesses—especially small and mid-sized ones—can harness the power of machine learning without hiring dedicated data scientists. Furthermore, the democratization of ML through AutoML contributes to faster innovation and better decision-making across industries.
6. Reinforcement Learning Powers Robotics and Logistics
Reinforcement learning (RL) has made significant strides, especially in fields that involve complex decision chains. In 2025, RL is widely used in robotics, supply chain optimization, and even autonomous vehicles.
What sets RL apart is its ability to learn from trial and error. For example, robots in manufacturing plants can now adjust their movements based on real-time feedback, improving both speed and accuracy. Similarly, logistics companies are using reinforcement learning to create smarter routing systems, which reduce costs and improve delivery times.
7. Data-Centric AI Gains Momentum
Traditionally, machine learning innovation focused on model architecture. However, a growing machine learning trend emphasizes the importance of high-quality data. Data-centric AI focuses on improving the training data itself—making it cleaner, more accurate, and more representative.
Companies are now investing in data labeling platforms, annotation tools, and validation pipelines to ensure their datasets are optimized. This shift enhances model performance and reduces the risk of algorithmic bias. Consequently, data quality is emerging as the new competitive advantage in the ML ecosystem.
8. Federated Learning Enhances Privacy
In an era of increasing data privacy concerns, federated learning stands out as a transformative innovation. Rather than sending sensitive data to a central server, federated learning allows models to train locally on users’ devices.
This approach not only protects user privacy but also reduces data transfer costs. It’s no surprise that tech giants are adopting federated learning for mobile applications, especially in areas like predictive typing, recommendation engines, and voice assistants.
9. Hybrid Models Combine ML with Symbolic AI
While deep learning excels at pattern recognition, symbolic AI handles logic and rules-based reasoning. In 2025, machine learning trends highlight the growing interest in hybrid models that combine both approaches.
These models deliver the best of both worlds—deep learning’s flexibility and symbolic AI’s interpretability. Applications range from financial compliance systems to natural language understanding, where complex rules must be applied to unstructured data.
10. Green AI and Sustainable Machine Learning
Finally, the environmental impact of training large ML models has come under scrutiny. Consequently, “green AI” has become a crucial trend in 2025. Developers are now optimizing algorithms for energy efficiency, reducing computational waste, and using renewable energy sources for training.
Some companies are even quantifying the carbon footprint of their ML models and choosing architectures with lower environmental costs. As climate change concerns grow, sustainability will remain a vital consideration in future machine learning projects.
Final Thoughts
The machine learning trends of 2025 reflect both the maturity and expanding influence of this technology. As real-time AI, ethical considerations, and industry-specific applications grow in importance, businesses must stay ahead of the curve. Fortunately, with the rise of AutoML, federated learning, and sustainable practices, ML is becoming more accessible and responsible than ever before.
By embracing these trends, organizations can unlock unprecedented innovation, drive better outcomes, and remain competitive in an increasingly data-driven world.